Unlocking Tesla's Future: How Automated Data Labeling Will Transform Self-Driving Cars
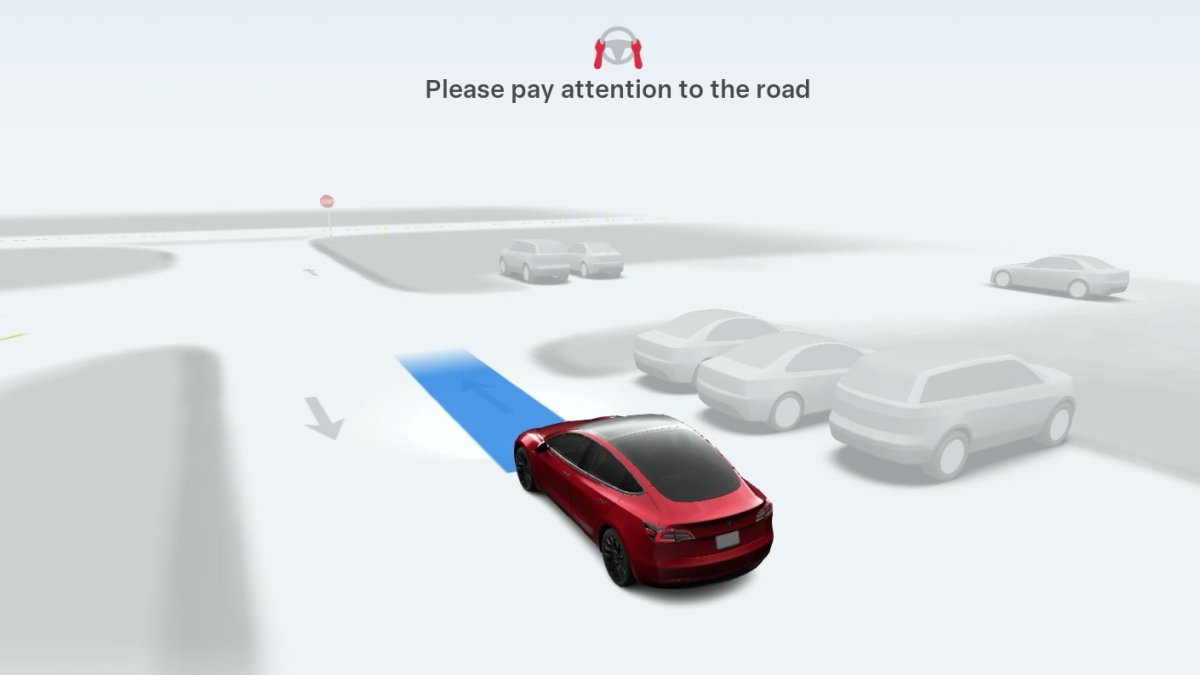
As we stand on the brink of a new era in automotive technology, Tesla continues to lead the charge towards fully autonomous driving with its innovative approach to data labeling for Full Self-Driving (FSD) systems. At the heart of this transformation lies Tesla's groundbreaking patent, WO2024073033A1, which outlines an advanced system designed to automate the once labor-intensive process of labeling vast amounts of driving data. This technology is not just a technical upgrade; it has the potential to revolutionize how artificial intelligence (AI) is trained in the automotive sector.
Tesla’s automated data labeling system offers a remarkable solution to one of the most time-consuming and error-prone tasks in developing AI models. Traditionally, human reviewers meticulously sift through hours of video footage, categorizing and tagging data for training purposes. This manual process is resource-heavy, slow, and prone to mistakes, making it a prime candidate for automation.
Understanding the Challenge of Data Labeling
Training an advanced AI system such as Tesla's FSD involves processing and labeling enormous collections of data derived from Tesla vehicles on the road. The challenge lies in ensuring that the data is correctly labeled, which is essential for teaching the AI to understand and navigate its surroundings. The traditional method of manual data labeling not only consumes an excessive amount of time and financial resources, but it is also subject to human error, a factor that can compromise the effectiveness of AI learning.
The Promise of Tesla's Automated System
Enter Tesla's automated solution—a model-agnostic system engineered to enhance the data labeling process. Drawing from the wealth of data gathered by Tesla’s ever-expanding fleet, this innovative setup utilizes advanced 3D modeling technology to facilitate automated labeling across a range of AI models. While the focus might be on FSD, the implications of such a system stretch beyond just driving technology, affecting numerous AI applications.
A Closer Look at the Process
The automated labeling system operates through a streamlined three-step approach designed to efficiently gather and implement new data. Let's break down each of these steps:
1. High-Precision Mapping
The first step involves constructing a highly accurate 3D map depicting the environment using data collected from multiple Tesla vehicles equipped with sophisticated sensors including cameras and radar. Think of it as creating a digital twin of the real world; this mapping forms the foundation of simulations that Tesla employs to rigorously test and refine its FSD technology. As more data is processed, the 3D model continuously enhances its accuracy, leading to better synthetic data generation for training purposes.
2. Multi-Trip Reconstruction
To capture the dynamic components of real-world environments, the system incorporates data from countless multiple trips through the same areas. This capability allows the AI to monitor constant variables, recognize moving objects, and track their behavior within a constantly shifting landscape. The result is a rich, interactive 3D model that reflects the complexities of traffic, pedestrians, and other environmental factors, paving the way for superior AI comprehension.
3. Automated Labeling
Once a detailed 3D model is established, it serves as the cornerstone for automated data labeling. As a Tesla vehicle encounters new scenes, the real-time data captures information and cross-references it against the intricate 3D model. Through this comparison, the system can automatically identify and label crucial features such as objects, lane markings, and directional signage in newly captured data.
The Advantages of Automation
Embracing automated data labeling presents significant benefits for Tesla in its pursuit of AI-driven technology:
- Efficiency: The automation process minimizes the time and resources required to prepare training data, which ultimately accelerates development cycles and allows Tesla to harness larger datasets for improved AI training.
- Scalability: This system can accommodate vast datasets derived from millions of driving miles logged by the Tesla fleet, continually enriching the FSD environment with more precise 3D modeling and, consequently, enhancing automated labeling.
- Accuracy: By reducing human error, automated labeling improves the quality and consistency of labeled data, leading to the development of robust and reliable AI models. Although humans will continue to review data at intervals, their primary function will be to catch anomalies rather than engage in the bulk of labeling work.
Wider Applications Beyond FSD
While the implications for FSD are significant, Tesla's automated labeling technology has broader applications across the AI spectrum. Among these applications include:
- Object Detection: Distinguishing and categorizing various objects within the vehicle's environment, such as other vehicles, pedestrians, and traffic signs.
- Kinematic Analysis: Modeling motion patterns and behavior of objects, providing insight into potential hazards and enhancing the vehicle’s predictive capabilities.
- Shape Analysis: Recognizing and discerning the forms and structures of objects, even if obscured or viewed from unconventional angles.
- Occupancy Detection: Assessing space constraints, identifying free spots, and understanding the different road surfaces.
Conclusion: The Path Forward
In essence, Tesla’s automated data labeling system stands as a monumental leap towards a future of enhanced AI development. By leveraging its expansive vehicle fleet and innovative 3D mapping technology, Tesla has birthed a self-learning system capable of evolving continuously with the acquisition of new data. This innovation envisions a landscape where self-driving vehicles autonomously label and interpret their environment, driving us headlong into a future laden with limitless possibilities. As Tesla forges ahead, the world watches eagerly for what lies ahead in the realm of autonomous transport.